GreenML
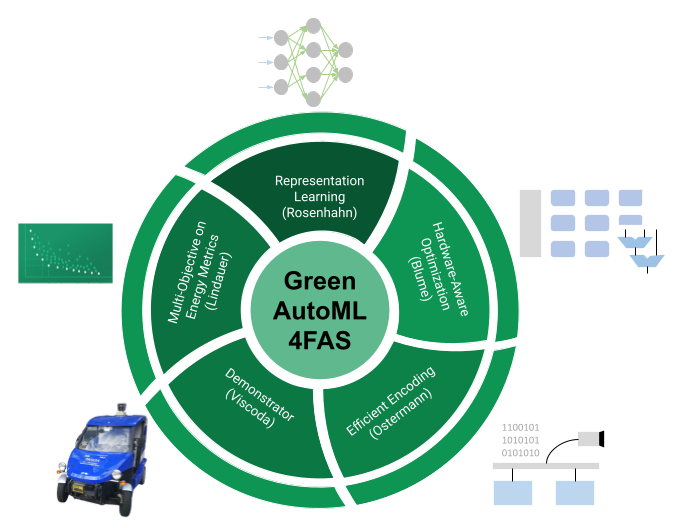
Led by: | Prof. Dr.-Ing. habil H. Blume |
Team: | Matthias Lüders |
Year: | 2023 |
Duration: | 2023-2026 |
The project "GreenML" aims to exemplify a holistic AI design process by the highly efficient and resource-optimized implementation of essential FAS functions like object detection, object classification, and scene contextualization on particular hardware. Deep Learning (DL) has become a central approach for modern AI applications. Even though energy-efficient DL has become a target in research, currently isolated solutions are often created that do not unleash the full potential for resource-efficient AI. In this project, we will focus on a holistic approach: from hardware to efficient coding and transfer of data and models to dynamic and resource-adaptive software to enable multi-criteria optimization of all facets of an AI-enabled system. As an example, we demonstrate the potential of this approach using the scenario of a modern driver assistance system (FAS). With about 67 million registered vehicles and increased e-mobility, saving required energy by combining efficient algorithms, communication, and hardware is urgently needed. Our "Green Assisted Driving" project addresses different energy consumption, safety, and flexibility metrics. The consortium combines low-power hardware, learning of efficient representations from large data sets, hyperparameter optimization, and network design using AutoML, as well as methods of transfer learning, semi-supervised learning, and network pruning to prototype highly efficient and dynamically controllable models on a FAS. and demonstrate the savings potential of a holistic approach.